Researchers from the University of Wisconsin-Madison and Microsoft Research have developed improved baselines for visual instruction tuning models that achieve state-of-the-art performance across 11 benchmarks.
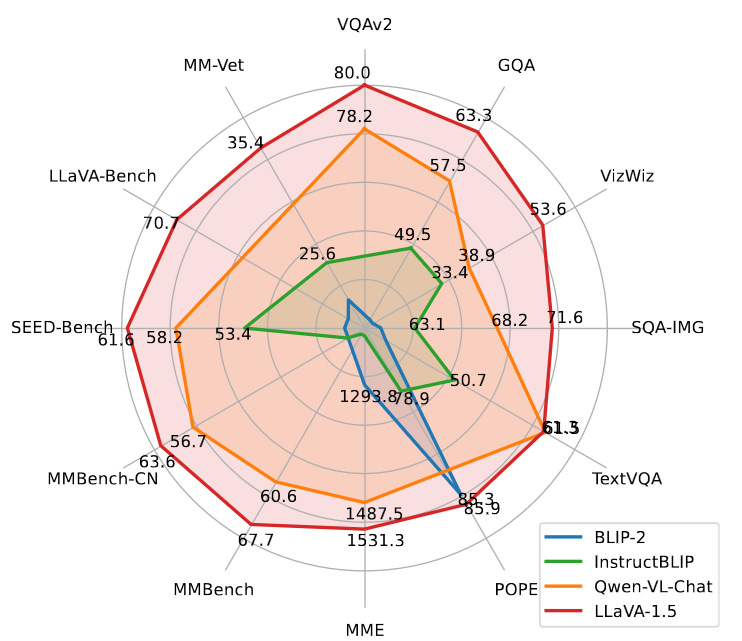
In their technical report “Improved Baselines with Visual Instruction Tuning“, the authors make simple modifications to the LLaVA model architecture that lead to significant gains. The key changes include using a two-layer MLP rather than linear connector between the visual encoder and language model, and incorporating academic task-oriented VQA data with clear response formatting prompts.
With these tweaks, the new LLaVA-1.5 model achieves top results on a diverse set of 12 evaluation benchmarks while using orders of magnitude less training data than comparable models like InstructBLIP and Qwen-VL.
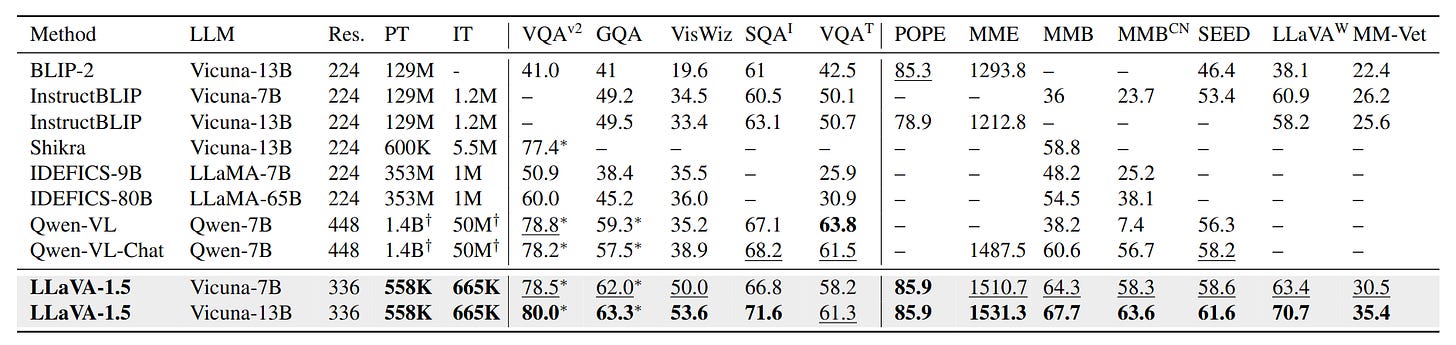
The authors attribute the strong performance to the power and efficiency of LLaVA’s full image patch design and transformer architecture. Although visual resampling methods can reduce computational costs, LLaVA converges faster and generalizes better with less data.
By establishing reproducible state-of-the-art baselines, this work makes large multimodal model research more accessible. The ability to train top-tier models without massive datasets or resources lowers the barrier for future open-source development.
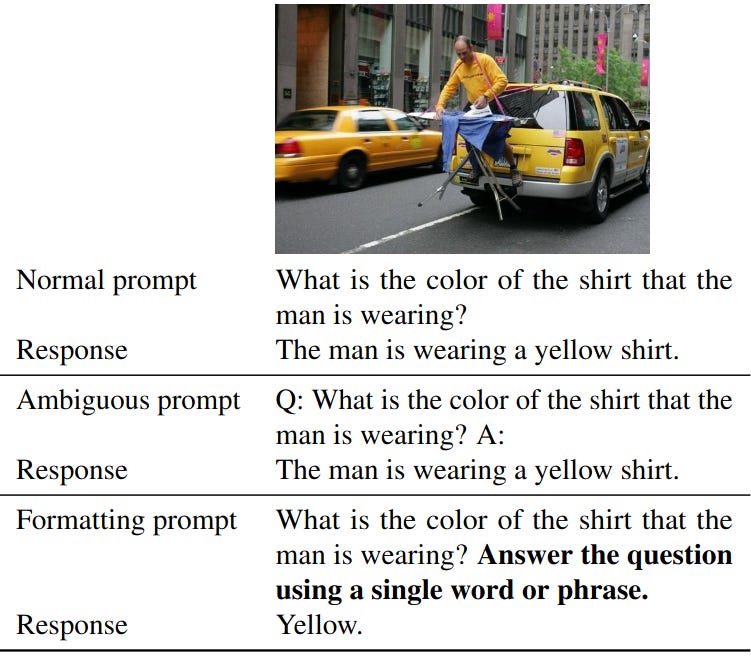
Looking forward, visual instruction tuning seems to have more impact on multimodal understanding than pretraining alone. However, limitations around multi-image processing, problem solving, and hallucination remain. If these improved baselines can be scaled up responsibly, they may one day power real-world assistive applications.